Throughout this unit on incomplete information, we have looked at rather simple games of incomplete information that do not involve the parties learning anything beyond their own preference type. However, many situations involving incomplete information involve an actor having a prior belief and then receiving a private signal that gives them more information. A similar type of situation will be at the forefront of next unit’s games, where players with private information take actions that their opponents can learn from. We do not yet know how to handle these situations.
On a seemingly unrelated note, we have also been using Bayesian Nash equilibrium as our solution concept. The Nash equilibrium part of the name is straightforward—it traces back to the Nash equilibrium from complete information settings. The Bayesian part has not yet had an explanation.
In fact, these two things tie together. The Bayesian part of the name comes from Thomas Bayes, an 18th Century statistician. His main contribution was in the correct way to update beliefs when encountering new information. This is known as Bayes’ rule, which we need to implement to solve the new types of strategic scenarios.
Takeaway Points
- Suppose we know the ex ante likelihood that A is true. We also know that conditional on A being true what the likelihood that B is true. And we further know that conditional on A being false what the likelihood that B is true. If we observe whether B is true, then we can use this information to calculate an updated belief about whether A is true.
- We call this a posterior belief. This contrasts with the original belief, which we describe as a prior belief.
- A common example of this is in medical testing. Suppose from historical trends we know what the likelihood someone has cancer is. There is a test that detect it. However, that test is not perfectly reliable. Thus, someone who tests negative might actually have cancer. Nevertheless, the result of the test provides a window into the individual’s health, and we should use that information to form a new belief about the individual. Bayes’ rule allows us to do this.
- Formally, the probability of A given B is the ex ante probability A is true times the probability of observing B given that A is true, divided by the sum of that plus the probability that A is false times the probability of observing B given that A is false.
- Another way to think about the calculation is to put the specific pathway of getting to B that you want to measure in the numerator. Then put all the different pathways to B in the denominator, including the way you want.
- If done correctly, the resulting quantity is always a probability. Moreover, it is the updated, posterior belief that A is true given the new information.
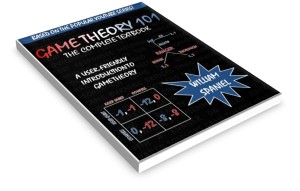